Matthias Ehrhardt
Das Geheimnis hinter der schnellen MRT
Was ist 'Compressed Sensing' ?
|
 |
Materialien für Interessierte zum Vortrag am
Die Zielgruppe sind Schüler ab der 11. Klasse.
|
 |
|
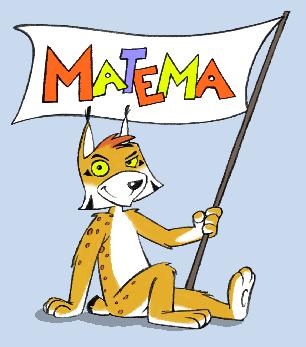
Das MATEMA-Logo (ein Luchs, copyright by Ulf Grenzer)
Beschreibung
Eine
Kernspintomografie, auch Magnetresonanztomografie (MRT) genannt, ist ein
bildgebendes Untersuchungsverfahren mit Hilfe eines starken Magnetfelds und eines
Computers. Moderne MRT-Verfahren sind Dank angewandter Mathematik deutlich schneller, so dass der Arzt
(z.B. nach einem Unfall) nicht so lange auf die medizinischen Schnittbilder warten muss.
Auch Patienten, die unter Klaustrophobie in den MRT-Geräten leiden, müssen nicht mehr so lange darin liegen bleiben.
In diesem Vortrag
werden die mathematischen Hintergründe dieses schnellen bildgebenden Verfahren erläutert; es basiert auf
spärlichen Signalen, Wavelets und der komprimierten Abtastung ('compressed sensing').
Es ist ein weiteres
Beispiel wie angewandte Mathematik (unbemerkt) in unserem Alltag steckt.

Das MATEMA-Logo (ein Luchs, copyright by Ulf Grenzer)
Referenzen für den Vortrag
- M. Aharon, M. Elad, A.M. Bruckstein,
The K-SVD: An algorithm for designing of overcomplete dictionaries for sparse representation,
IEEE Trans. Signal Proc. 54 (2006), 4311-4322.
- C.A. Baron, N. Dwork, J.M. Pauly, D.G. Nishimura,
Rapid compressed sensing reconstruction of 3D non-Cartesian MRI,
Magn. Reson. Med. 79(5) (2018), 2685-2692.
- L. Borup, R. Gribonval, M. Nielsen,
Beyond coherence: Recovering structured time-frequency representations,
Appl. Comput. Harmon. Anal. 14 (2008), 120-128.
- J. Bourgain, S. Dilworth, K. Ford, S. Konyagin, D. Kutzarova,
Explicit constructions of rip matrices and related problems,
Duke Math. J. 159 (2011), 145-185.
- B. Boufounos, G. Kutyniok, H. Rauhut,
Sparse recovery from combined fusion frame measurements,
IEEE Trans. Inform. Theory 57 (2011), 3864-3876.
- A.M. Bruckstein, D.L. Donoho, A. Elad,
From sparse solutions of systems of equations to sparse modeling of signals and images,
SIAM Rev. 51 (2009), 34-81.
- E.J. Candès,
The restricted isometry property and its implications for compressed sensing,
C. R. Acad. Sci. I 346 (2008), 589-592.
- E.J. Candès, Y.C. Eldar, D. Needell, P. Randall,
Compressed Sensing with Coherent and Redundant Dictionaries,
Appl. Comput. Harmon. Anal. 31 (2011), 59-73.
- E.J. Candès, B. Recht,
Exact matrix completion via convex optimization,
Found. of Comput. Math. 9 (2008), 717-772.
- E. Candès, J. Romberg, T. Tao,
Robust uncertainty principles: Exact signal reconstruction from highly incomplete Fourier information,
IEEE Trans. Inform. Theory 52 (2006), 489-509.
- P.G. Casazza, G. Kutyniok,
Finite Frames: Theory and Applications,
Birkhäuser, Boston, 2012.
- P.G. Casazza, G. Kutyniok, S. Li,
Fusion Frames and Distributed Processing,
Appl. Comput. Harmon. Anal. 25 (2008), 114-132.
- S.S. Chen, D.L. Donoho, M.A. Saunders,
Atomic decomposition by basis pursuit,
SIAM J. Sci. Comput. 20 (1998), 33-61.
- A. Cohen, W. Dahmen, R. DeVore,
Compressed sensing and best k-term approximation,
J. Am. Math. Soc. 22 (2009), 211-231.
- I. Daubechies,
Ten Lectures on Wavelets,
SIAM, Philadelphia, 1992.
- I. Daubechies, M. Defrise, C. De Mol,
An iterative thresholding algorithm for linear inverse problems with a sparsity constraint,
Comm. Pure Appl. Math. 57 (2004), 1413-1457.
- R. DeVore,
Deterministic constructions of compressed sensing matrices,
J. Complexity 23 (2007), 918-925.
- D.L. Donoho,
Compressed sensing,
IEEE Trans. Inform. Theory 52 (2006), 1289-1306.
- D.L. Donoho, M. Elad,
Optimally sparse representation in general (nonorthogonal) dictionaries via l1 minimization,
Proc. Natl. Acad. Sci. USA 100 (2003), 2197-2202.
- D.L. Donoho, M. Elad, V. Temlyakov,
Stable recovery of sparse overcomplete representations in the presence of noise,
IEEE Trans. Inform. Theory 52 (2006), 6-18.
- D.L. Donoho, X. Huo,
Uncertainty principles and ideal atomic decomposition,
IEEE Trans. Inform. Theory, 47 (2001), 2845-2862.
- D.L. Donoho, G. Kutyniok,
Microlocal analysis of the geometric separation problem,
Comm. Pure Appl. Math. 66 (2013), 1-47.
- D.L. Donoho, A. Maleki, A. Montanari,
Message passing algorithms for compressed sensing,
Proc. Natl. Acad. Sci. USA 106 (2009), 18914-18919.
- D.L. Donoho, P.B. Starck,
Uncertainty principles and signal recovery,
SIAM J. Appl. Math. 49 (1989),906-931.
- D.L. Donoho, J. Tanner,
Neighborliness of Randomly-Projected Simplices in High Dimensions,
Proc. Natl. Acad. Sci. USA 102 (2005), 9452-9457.
- D.L. Donoho, J. Tanner,
Sparse Nonnegative Solutions of Underdetermined Linear Equations by Linear Programming,
Proc. Natl. Acad. Sci. USA 102 (2005), 9446-9451.
- D.L. Donoho, J. Tanner,
Observed universality of phase transitions in high-dimensional geometry,
with implications for modern data analysis and signal processing,
Philos. Trans. Roy. Soc. S.-A 367 (2009), 4273-4293.
- M. Elad,
Sparse and Redundant Representations,
Springer, New York, 2010.
- M. Elad, A.M. Bruckstein,
A generalized uncertainty principle and sparse representation in pairs of bases,
IEEE Trans. Inform. Theory 48 (2002), 2558-2567.
- M. Elad, J.-L. Starck, P. Querre, D.L. Donoho,
Simultaneous cartoon and texture image inpainting using morphological component analysis (MCA),
Appl. Comput. Harmon. Anal. 19 (2005), 340-358.
- Y.C. Eldar, G. Kutyniok (eds.),
Compressed Sensing: Theory and Applications,
Cambridge University Press, 2012.
- L. Feng, S. Coppo, D. Piccini, et al.,
5D whole-heart sparse MRI,
Magn. Reson. Med. 79(2) (2018), 826-838.
- M.A.T. Figueiredo, R.D. Nowak, S.J. Wright,
Gradient projection for sparse reconstruction:
Application to compressed sensing and other inverse problems,
IEEE J. Sel. Top. Signa. 1 (2007), 586-597.
- S. Foucart,
A note on guaranteed sparse recovery via l1-minimization,
Appl. Comput. Harmon. Anal. 29 (2010), 97-103.
- A.C. Gilbert, M.J. Strauss, R. Vershynin,
One sketch for all: Fast algorithms for Compressed Sensing,
in: Proc. 39th ACM Symp. Theory of Computing (STOC), San Diego, CA, 2007.
- B. Grünbaum,
Convex polytopes,
Graduate Texts in Mathematics 221, Springer, New York, 2003.
- M. Herman, T. Strohmer,
High Resolution Radar via Compressed Sensing,
IEEE Trans. Signal Proc. 57 (2009), 2275-2284.
- M.A. Iwen,
Combinatorial Sublinear-Time Fourier Algorithms,
Found. of Comput. Math. 10 (2010), 303-338.
- P. Jain, A. Tewari, I.S. Dhillon,
Orthogonal Matching Pursuit with Replacement,
in: Proc. Neural Inform. Process. Systems Conf. (NIPS), 2011.
- G. Kutyniok,
Clustered Sparsity and Separation of Cartoon and Texture,
SIAM J. Imaging Sci. 6 (2013), 848-874.
- G. Kutyniok, D. Labate,
Shearlets: Multiscale Analysis for Multivariate Data,
Birkhäuser, Boston, 2012.
- G. Kutyniok, W.-Q Lim,
Compactly supported shearlets are optimally sparse,
J. Approx. Theory 163 (2011), 1564-1589.
- G. Kutyniok, W.-Q Lim,
Image separation using shearlets,
in: Curves and Surfaces (Avignon, France, 2010),
Lecture Notes in Computer Science 6920, Springer, 2012.
- Y. Lu, M. Do,
Sampling signals from a union of subspaces,
IEEE Signal Proc. Mag. 25 (2008), 41-47.
- M. Lustig, D. Donoho, J.M. Pauly,
Sparse MRI: The application of compressed sensing for rapid MR imaging,
Magn. Reson. Med. 58(6) (2007), 1182-1195.
- S.G. Mallat,
A wavelet tour of signal processing: The sparse way,
Academic Press, Inc., San Diego, CA, 1998.
- S.G. Mallat, Z. Zhang,
Matching pursuits with time-frequency dictionaries,
IEEE Trans. Signal Proc. 41 (1993), 3397-3415.
- M. Mishali, Y.C. Eldar, A. Elron.
Xampling: Signal Acquisition and Processing in Union of Sub spaces,
IEEE Trans. Signal Proc. 59 (2011), 4719-4734.
- S. Muthukrishnan,
Data Streams: Algorithms and Applications,
Now Publishers, Boston, MA, 2005.
- S. Nam, M.E. Davies, M. Elad, R. Gribonval,
The Cosparse Analysis Model and Algorithms,
Appl. Comput. Harmon. Anal. 34 (2013), 30-56.
- D. Needell, J.A. Tropp,
CoSaMP: Iterative signal recovery from incomplete and inaccurate samples,
Appl. Comput. Harmon. Anal. 26 (2008), 301-321.
- D. Needell, R. Vershynin,
Uniform Uncertainty Principle and signal recovery via Regularized Orthogonal Matching Pursuit,
Found. of Comput. Math. 9 (2009), 317-334.
- Y.C. Pati, R. Rezaiifar, P.S. Krishnaprasad,
Orthogonal matching pursuit: Recursive function approximation with applications to wavelet decomposition,
in: Proc. of the 27th Asilomar Conference on Signals, Systems and Computers 1 (1993), 40-44.
- H. Rauhut, K. Schnass, P. Vandergheynst,
Compressed sensing and redundant dictionaries,
IEEE Trans. Inform. Theory 54 (2008), 2210-2219.
- J. Schoormans, Q. Zhang, B. Coolen, G. Strijkers, A. Nederveen,
Reconstruction of sparsely sampled Magnetic Resonance Imaging measurements with a convolutional neural network,
MIDL 2018, OpenReview.net.
- T. Strohmer, R.W. Heath,
Grassmannian frames with applications to coding and communication,
Appl. Comput. Harmon. Anal., 14 (2004), 257-275.
- J.A. Tropp,
Greed is good: Algorithmic results for sparse approximation,
IEEE Trans. Inform. Theory 50 (2004), 2231-2242.
- P. Walk, P. Jung,
Compressed Sensing: Applications to Communication and Digital Signal Processing,
Foundations in Signal Processing, Communications and Networking 20, Springer 2019.
- X. Weiyu, B. Hassibi,
Compressive Sensing over the Grassmann Manifold: a Unified Analytical Framework,
in: 46th Annual Allerton Conf. on Communication, Control, and Computing, 2008.
- G. Yang, S. Yu, H. Dong, et al.,
DAGAN: Deep De-Aliasing Generative Adversarial Networks for Fast Compressed Sensing MRI Reconstruction,
IEEE Trans. Medical Imaging 37(6) (2018), 1310-1321.
- B. Zhu, J.Z. Liu, S.F. Cauley, et al.,
Image reconstruction by domain-transform manifold learning,
Nature 555 (2018), 487-492.
Links:
- The Gauss Prize 2018: David Donoho