Matthias Ehrhardt
Wie man am schnellsten Fussballexperte wird
Machine Learning im Sport
|
 |
Materialien für Interessierte zum Vortrag am
Die Zielgruppe sind Schüler ab der 11. Klasse.
|
 |
|
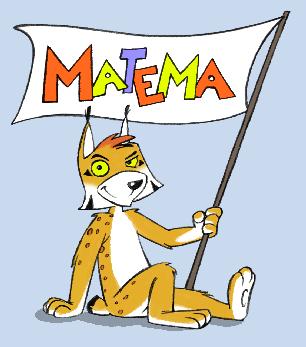
Das MATEMA-Logo (ein Luchs, copyright by Ulf Grenzer)
Beschreibung
Aus Anlass der Fussball-Weltmeisterschaft in Russland 2018 wollen wir den Fussball aus mathematischer Sicht betrachten.
Wir wollen in diesem Vortrag untersuchen, wie man Machine Learning (oder: Künstliche Intelligenz) einsetzen kann,
um Informationen über Spieler, Teams usw. zu gewinnen.
WM 2018: Deutschland scheidet als Gruppenletzter in Vorrunde aus, England gewinnt ein Elfmeterschießen. Selbst Fussballexperten werden noch
von den Ereignissen überrascht.
In diesem Vortrag werden wir die Grundzüge von Machine Learning erklären, und zeigen wie man damit (in Echtzeit, also
während das Spiel läuft) wichtige Informationen über einzelne Spieler (eigene und gegnerische) und über Spielstrategien
des Gegners gewinnen kann. Somit wird man ohne Vorkenntnisse sofort zum Fussballexperten und in Zukunft könnte theoretisch ein Computer die
Rolle eines Trainers übernehmen (bis auf die fehlende Empathie).
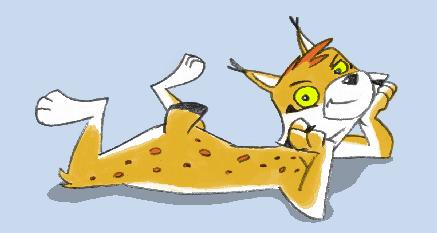
Das MATEMA-Logo (ein Luchs, copyright by Ulf Grenzer)
Referenzen für den Vortrag
- E. Bahceci,
High performance computing and visualization of football match results-from algorithms built up using methods from modeling complex systems,
Degree Project in Engineering Physics, Uppsala University, 2017, diva-portal.org.
- A. Brauchle,
Anwendung von Data-Mining-Technologien zu statistischen Auswertungen und Vorhersagen im Fußball,
Masterarbeit, Universität Hohenheim, 2016.
- J.M. Buldu, J. Busquets, J.H. Martínez,
Using network science to analyze football passing networks: dynamics, space, time and the multilayer nature of the game,
arXiv preprint, 2018.
- R. Maneiro Dios, M. Amatria Jiménez,
Polar Coordinate Analysis of Relationships With Teammates, Areas of the Pitch, and Dynamic Play in Soccer: A Study of Xabi Alonso,
Frontiers in psychology, 2018.
- R.M. Dios, MA Jiménez, J.E.M. García,
Análisis observacional de las relaciones interlíneas de la Selección Española de Fútbol,
mediante coordenadas polares,
Cuadernos de Psicología, 2018.
- T. Harkness
Big Data: Does Size Matter?,
Bloomsbury Sigma, 2016.
- T. Narizuka, Y. Yamazaki,
Classification algorithm for formations in football games,
arXiv preprint arXiv:1805.07525, 2018.
- J.A. Ruiz-Vanoye, O. Díaz-Parra, A. Fuentes-Penna et al.,
Motivation Index to Improve the Soccer Performance,
Int. J. Combin. Optim. Probl. Inform., Vol. 8, No. 3, 2017, pp. 45-57.
- D. Sumpter,
Soccermatics: Mathematical Adventures in the Beautiful Game,
Bloomsbury Sigma, 2017.